[/vc_column][/vc_row]
In system level, we focus on realizing smart manufacturing systems.
We utilize automatic optical inspection (AOI) and machine learning (ML), training artificial intelligence (AI) models, predicting off-line electrical performance of microelectronic components from their in-line pattern integrities. This topical research starts from linear pattern recognition, and supports nonlinear but symmetrical patterns (such as circles and ellipses). Special pattern deteriorations in printed circuits were observed, which can be scientifically judged regardless of evaluation moment and observer. With algorithm advancement, asymmetrical patterns (but mathematically describable) such as various spirals for antenna applications are also successfully evaluated.
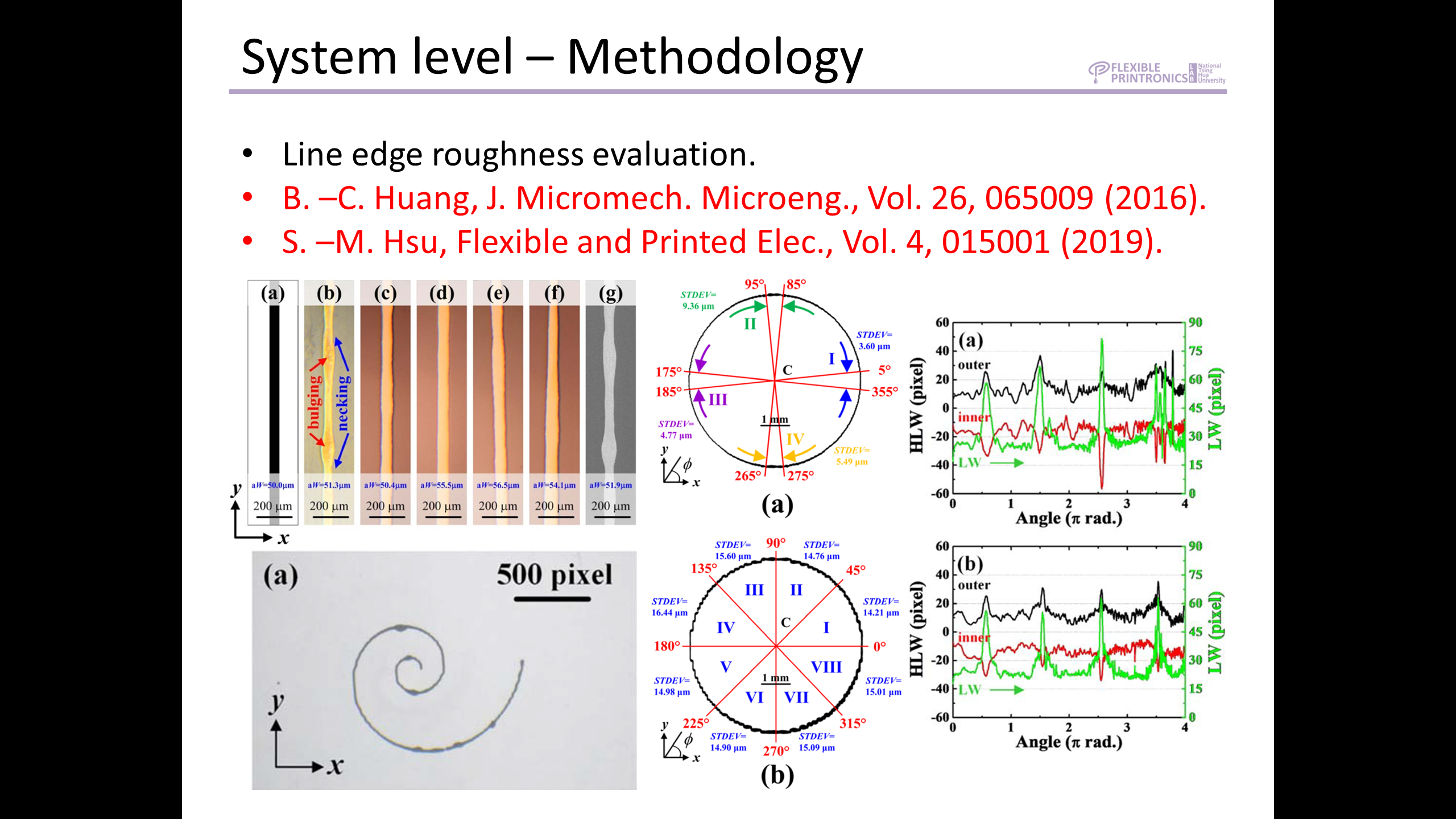
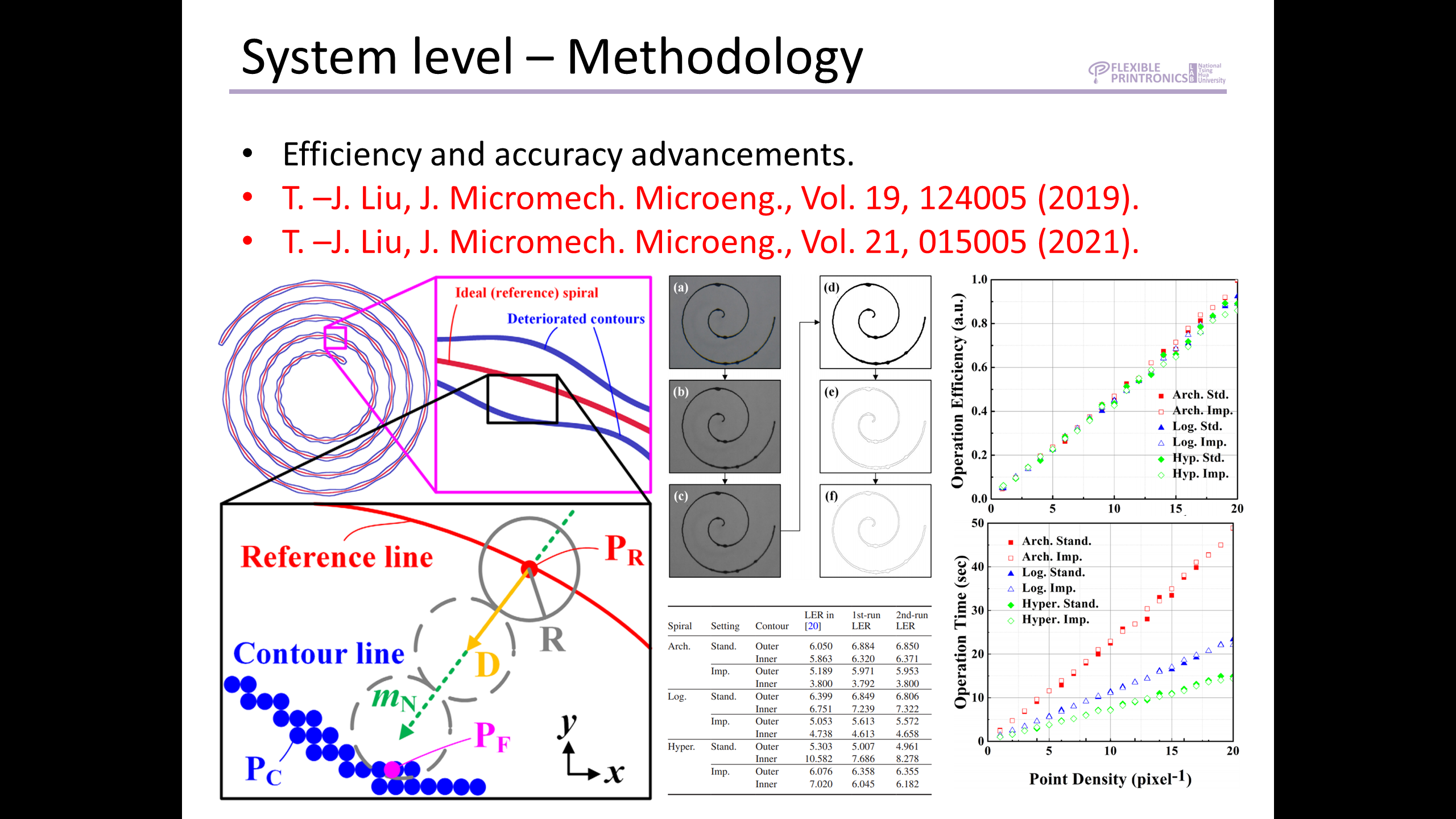
With further advancement on algorithm efficiency and accuracy, six features were evaluated by the training group of samples for different labels through ML. Results from the testing group of samples indicated that the frequency of the antenna not only shifted with deteriorated patterns but also the electrical deviation was successfully predicted by the established AI. This work presents a smart manufacturing solution that off-line electrical behaviors of microelectronic components can be predicted by their in-line fabrication characteristics (such as passive capacitance and active frequency), saving cost and time in production lines. With the developed AOI, analysis, and prediction software of graphical user interface (GUI), these results can also be applied to other industries as an advanced defect inspection method.
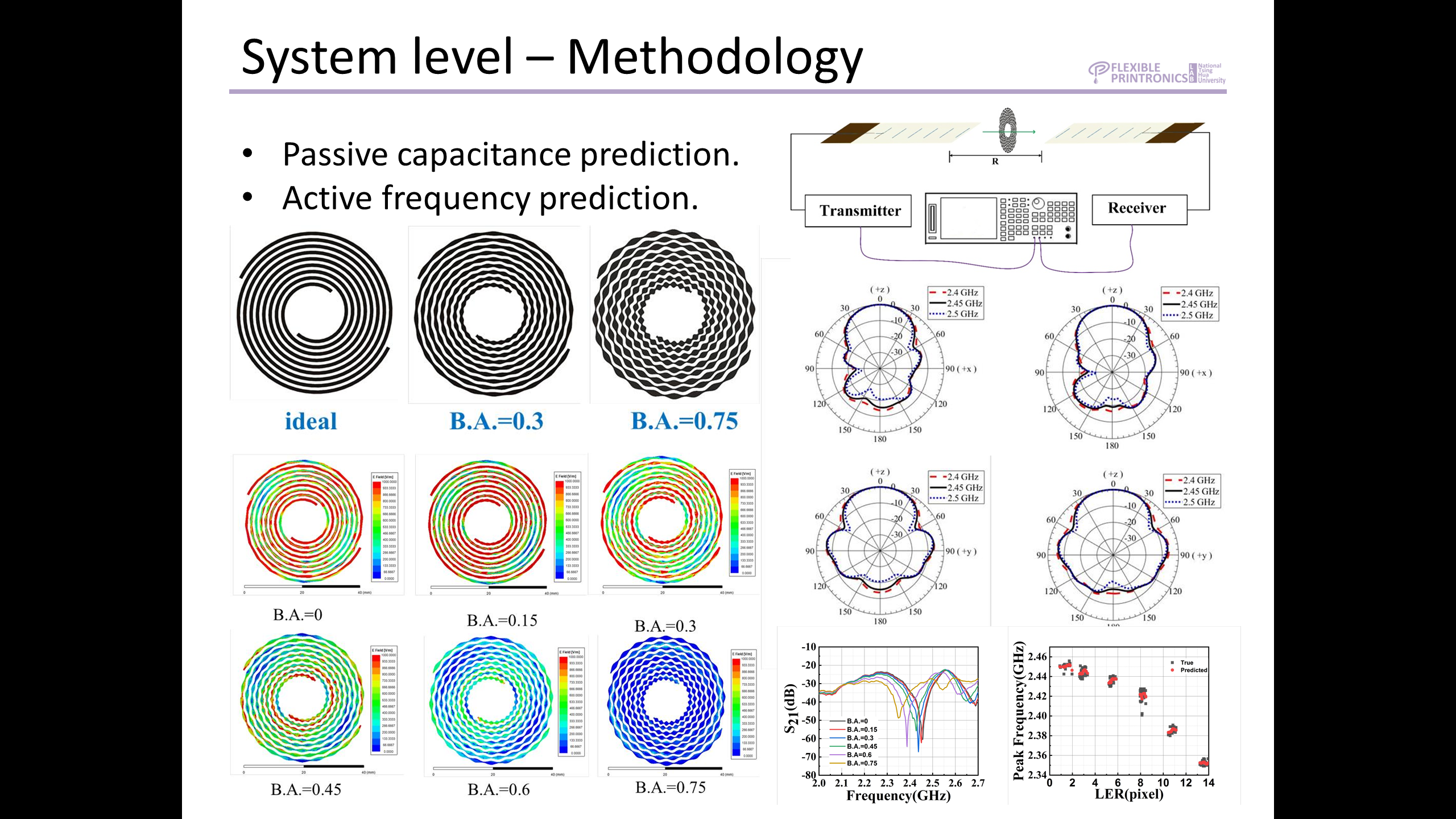
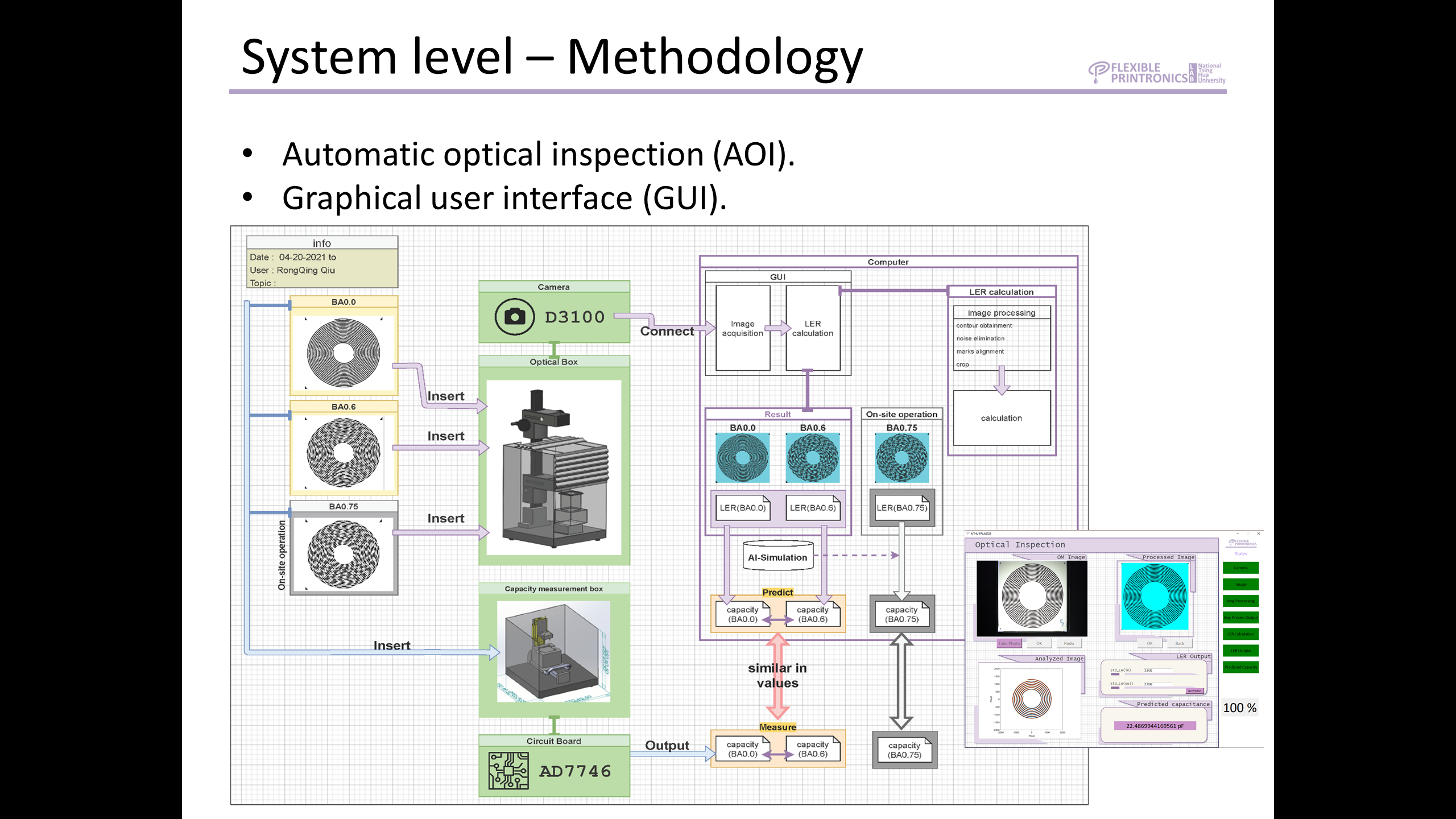